The Economics of Oppenheimer
Do you think the Oppenheimer movie could trigger a blog post about the economics of science? I believe it already has.
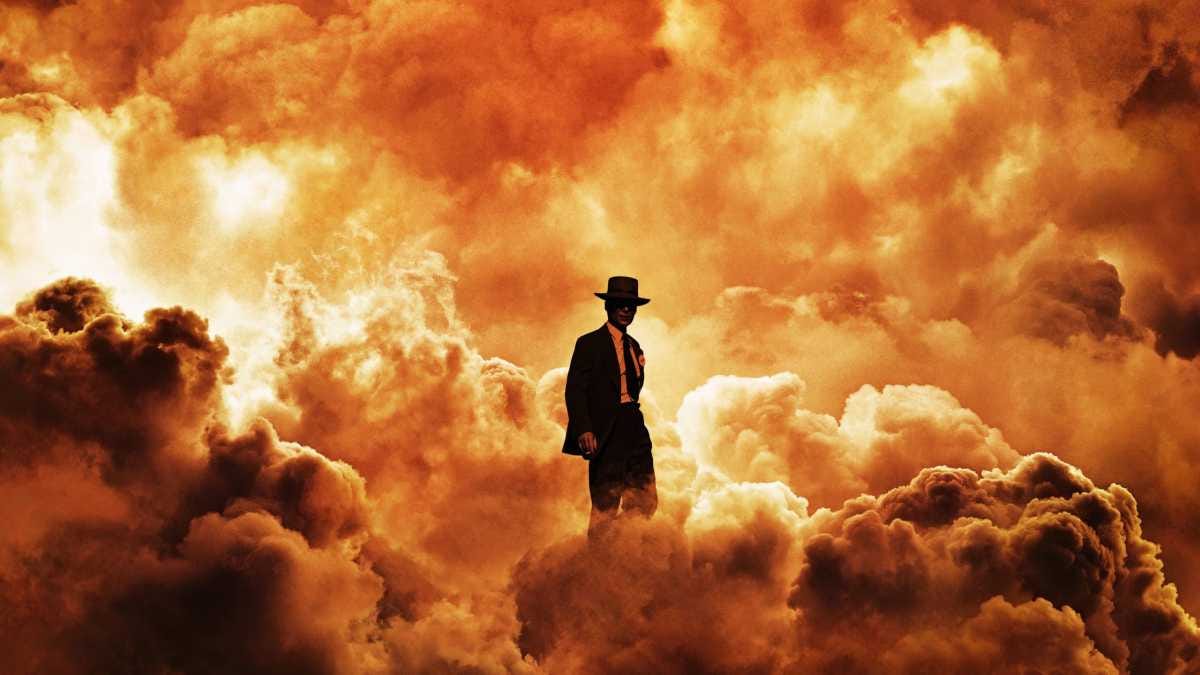
Oppenheimer ended up winning Best Picture, surprising neither awards watchers, casual film bros, nor dads who were disappointed by Napoleon. I saw Oppenheimer and I liked it. I watched it twice, even. And because Julius Robert “Oppie” Oppenheimer was an interesting figure, I started to wonder: what can economics tell us about science? Besides being dismal at it, I mean.
Death, the destroyer of worlds
[Oppenheimer] won nothing and acquiesced to everything.
American Prometheus
Oppenheimer follows, in somewhat of a chronological manner, the life of physicist J Robert Oppenheimer, mainly through three time periods: his career, from being a student at Cambridge to detonating the first nuclear bomb at Los Alamos, the 1954 hearing resulting in the ultimate denial of his security clearance to work for government, and the 1959 failed confirmation hearing of Oppenheimer’s colleague Lewis Strauss to the position of Secretary of Commerce.
If you’ll have learned anything from the Barbie movie, it’s that life only has one ending, and Oppenheimer’s life is relatively straightforward: he goes to Cambridge and later Gottingen, where he meets such luminaries as Niels Bohr and Werner Heisenberg; he goes to work at UC Berkeley, starting a quantum physics program. He gets drafted into the Manhattan Project but only at the cost of renouncing his communist friends, and succeeds in bringing it to fruition through the Trinity Test.
However, Oppenheimer’s political beliefs and his lack of commitment to further nuclear armament (and to, in fact, the opposite) begins to splash back - he starts losing standing among the DC elite for not being a fanatical anticommunist, which results in Lewis Strauss, a conservative nuclear hawk who works with him at what would become the Energy Department later, deciding to take Oppenheimer down. He proceeds to put together a sham hearing to discredit Oppenheimer, where he loses his security clearance and therefore his credibility.
Oppenheimer’s loss of a security clearance completely derails his career, which never recovers. Lewis Strauss, meanwhile, is ascendant in Republican circles, and is nominated as Secretary of Commerce (a low stakes, not especially relevant position) in 1959. However, Oppenheimer comes back to haunt Strauss, who becomes one of only a handful of Cabinet nominees to ever lose a confirmation vote - because of the high opinion scientists have of Oppenheimer (and, conversely, how much they dislike him).
While the science part may be interesting and all, the movie has to deal with politics, because the development of nuclear weapons radically altered like, the world. It was important not only to show how devastating Oppenheimer’s creation was, but also what kind of people to whom he gave that sort of power: petty, vindictive divas like Lewis Strauss (or insensitive types like Harry Truman). Oppenheimer’s cardinal flaw was always his lack of interest in having a position on literally anything happening around him - something his less well-intentioned colleagues made up for.
Oppenheimer tries to deal with Oppie's guilt around creating a weapon that could destroy humanity. But how? Let’s start at the broadest level: war. Obviously a first argument is that war simply outweighs its costs in some realm, or that leaders are acting irrationally or dealing with irrational counterparties, or even that in some cases, the ruling elite wouldn’t be the one fighting. Also that countries might sense a war is inevitable, and want to strike first. But most of these options can’t really account for why countries can’t just make some sort of political deal. There are, largely speaking, there are two major reasons why: first, differences in information between other countries and your own country - one country says “they’ll never agree to such-and-such conditions” and decides the only possibility is war, even if the other country is actually willing to agree to those conditions - but it may claim it won’t, to secure better ones. The second reason is that maybe two states do actually reach a solution, but it’s not one they have any incentives to respect - if they both agree not to arm themselves and that’s that, they’ll probably end up arming themselves just in case.
In both cases, countries are incentivized to overstate their capacity to win a war and understate how averse they are to a war actually breaking out - which can actually lead to more war than just telling the truth, because truthfulness makes negotiations harder. The same general principle applies to nuclear war: countries don’t want to strike first, but if you promise not to strike first, you have the lower hand in any negotiations you’re involved in. So countries have to promise to strike first. One example of this dynamic can be this game, where people choose a number from 0 to 100 where whoever says the bigger number wins 100 bucks, but whatever number they add up to is the chance they have to both lose 10,000 dollars. Most people choose either 0, 50, or 100 - bad news. But you may actually say you’ll choose a big number to have an easier time winning with a small number, but instead you just end up with your opponent saying a really really big number - i.e. nuclear war.
A related conflict is what they call mutually assured destruction. If you have nuclear weapons, you need to have enough to destroy any other country, so that they don’t strike first to prevent you from wiping them out. The idea is simple: if either side gets too much more powerful than the other, it might tip the scales into nuclear war. This is basically what Oppenheimer’s enemies advocated for: to keep expanding America’s nuclear capacity to prevent Soviet dominance, which would lead to war (or communism). However, there are some issues: first, random mistakes may occur. Secondly, it’s possible that the other actor is not rational and will deploy nukes regardless of whether you have them, which forces you to play ball. This is, more or less, what Donald Trump was perceived to be doing regarding North Korea in 2018.
Camp El Alamo
While one may be under the impression that Robert Oppenheimer’s accomplishments in nuclear weaponry might be related to some sort of scientific breakthrough, but it wasn’t: nuclear fission, the concept powering the atomic bomb, was discovered before the war by German phycists. It also wasn’t any specifical feature of implementation: most of the relevant devices either already existed, or weren’t invented or expanded by Oppenheimer. Rather, his greatest strenght was as the core organizing force of El Alamo, the military base where the nuclear bomb was created. If El Alamo was a sort of summer camp for phycists (after all, they were having sex all the time), then Oppenheimer’s role was as its chief camp counselor.
General Groves and Oppenheimer’s idea to create a small location full of scientists was fairly smart, from an economics perspective. One can say that El Alamo was a purpose-made cluster, an area where an economic activity is concentrated, leading to large technical, cultural, or productive breakthroughs. For example, Los Angeles is home to the “Hollywood cluster”, New York to the “Wall Street cluster”, Seattle and San Francisco to high tech clusters. The exact economic forces that form them are complex, but needless to say, they make specific locations very economically significant. These clusters, particularly for scientific innovation, tend to move over time: from trxtile mills in 19th century Massachussetts electric plants in turn-of-the-century Cleveland, alongside well known examples like Boston, Palo Alto, or Detroit. A series of studies found that cities home to clusters are crucial for scientific advances, with surprising facts like mathematicians being less likely to cite physically distant work, or economists becoming more productive when working near other productive economists. One consequence of this, thus, is that whether cities can accomodate higher populations has profound implications for scientific research.
Of course, this leads us to questions of whether or not the specific organization of scientific production is important, a rather difficult query to answer. Back in the 40s, 50s, and 60s, most scientific innovation tended to take place in industrial labs: extremely large research facilities owned by a single organization, that performed a wide array of research. This research was later published, and its authors tried to angle for a patent. The reasons why this model was adopted were, largely, sky-high transaction costs for inter-firm scientific collaboration. Additionally, there were large positive externalities to be had from assembling a large team of experts under one roof: one could take a discovery straight from theory into consumer products.
This model, however, has largely disappeared: whereas in 1975 around 47% of patents were given to private companies, in 2006 only 6% were. This is mirrored by a decline in the number of scientists working for large companies, and in the size of companies that employ them. The big question is why this happened: firstly, it may have gotten harder to ensure that any specific discoveries be used to produce viable consumer products, for any number of reasons. Additionally, it might have not been sustainable to engage in basic research, which is extremely costly, especially when that research could be used by competitors afterwards. One interesting data point in this direction is that when Bell Labs was broken up in 1956, it was forced to give up rights over most of its patents. There is evidence that this move increased innovation, but only outside the telecomm sector: meaning that this might have disincentivized research directly (since Bell’s patents were already there), but also, by making attaining a patent that would ensure market dominance less appealing. Antitrust may have actually also had an opposite effect, since less stringent restrictions on mergers and acquisitions may have led to a model where small companies invent a product with the aim of being acquired by a larger company (sometimes simply so that the other company won’t compete with them). Lastly, the costs of acquiring and using another company’s technology are declining due to technological factors (i.e. the internet), meaning that simply put, mass communications may have doomed large industrial labs.
Modern scientific production is not without problems: a lot of findings aren’t reliable, many scientific findings are neither very useful in the real world nor rigorous enough, rates of scientific progress are declining (more on this later), and many scientific papers are not utilized properly. What can be done? Well, firstly, many have proposed increased public funding. A study has found that publicly funded R&D tends to be more fundamental to other work, be more “ahead of time” than alternatives, and be more likely to generate spillovers, particularly to smaller firms. However, increased funding isn’t a panacea: how research is allocated, what kind of research scientists are incentivized to do, and the kind of workload scientists are under are among the many problems of contemporary US scientific policy. The problems are, sadly, very complex.
Product of our environment
Previously, I mentioned that scientific progress is slowing down. What did I mean by this? The basic fact is that Total Factor Productivity growth, which is what economists use to measure technological changes, is slowing down. This has been happening for a couple of decades, and represents a break from other eras with big technological breakthroughs, but without wide-rangign wage implications.
Generally speaking, there are some main causes: the decline in population growth (which is linked to innovation and entrepreneurship), the decline in geographic mobility (tied to housing costs), the decline in the growth rate of educational attainment, and the shift from a goods-producing to a services producing economy (tied to something called Baumol’s cost disease). Other factors include shifts in worker skill and training, changes in how intangibles affect the economy, and different allocations of resources - for example, Italian productivity is so low due to low adoption of new technologies by companies, due to management incentives.
Now, there are two big caveats: the first is that the decline in TFP can be linked to “good” factors about family size, education, housing, etc. Secondly, TFP is pretty bad at measuring “technology”, mainly because it’s derived from very noisy economic data on GDP growth versus utilization estimates of labor and capital (the latter is pretty difficult to get right). And interpreting TFP to mean “technology” is pretty overgenerous to TFP, when it could include things like intangible improvements in the quality of inputs, or changes in the quality of products, or organizational methods. Plus, the Baumol’s story about services might not even be true, and instead improvements to the sector get caught up in hazy “quality” adjustments attributed to nobody. And the stuff about demographics and business dynamism might have to do with other regulatory changes that shift the age profile of innovators, and such. Shifts in how the benefits to new technologies are accrued may have occurred for the infomration era, too.
But there is one fact that cuts through this entire debate quite easily: scientific innovation is getting harder. Ideas are getting harder to find: bigger and bigger scientific teams, with higher and higher budgets, are required to produce the same amount of research. At the micro level, science is getting harder: papers have a growing number of authors and coauthors, research output is getting smaller, citation counts are growing, Nobel Prizes are awarded to older and older work, and fewer and fewer new papers are making it into the most cited papers of a given year. At the macro level, this means that maintaining a given level of technological output requires more and more resources, which is clearly not sustainable. This can be seen across a wide variety of fields, including more practical-minded ones like agriculture, and has even spread to economics.
What are the explanations? First, what’s called the “burden of knowledge”, or what economists might call “declining marginal returns”. Basically, when you know very little, it’s easy to learn more, but as the number of open questions shrink, the ones that remain have to be definitionally harder to solve. There is some evidence for this interpretation, but it can’t really explain the whole problem. Additionally, there could be a lack of diversity among inventors that is holding back new ideas from taking shape - more diverse teams are more productive and more successful, and a US randomized government program to aid new inventors in obtaining patents overwhelmingly benefitted women. Declining geographic mobility (once again, explained by housing costs) could also help explain part of it, since the location of institutions affects their output and because not having the right people in the right place has enormous costs for innovation. Cultural changes, especially within the sciences, may have affected the output and prodcutivity of scientists.
Another problem has to do with incentives for scientists and for publishing: scientists are incentivized to maximize the number of publications they put out, which drastically shpaes the shape of their research. Likewise, a focus on the number of citations per paper resulted in focusing on “safer” bets that are more likely to be smaller and less “significant” to the discipline. Likewise, there is a big divergence between universities and the private sector in what they research, with academics focusing on basic science (“what is a neutron” type questions) and the private sector lasering in on uses - a process that is not linear by any means, even if publicly funded R&D is largely a net positive for the private sector. Scientific publishing has become slow and time-consuming, resulting in lower output, without even (as stated above) ensuring usefunless or replicability of results.
One final point to make is that scientists are getting older, which is correlated with negative qualities of research: smaller, less important, and less disruptive to established ideas1. Part of this is caused by the fact that the labor force in general is getting smaller, part of it is the “burden of knowledge” problem requiring growing specialization, and part of it is a lack of retirements among older researchers. For example, the average age in the Manhattan Project was 29, and the modal age was 27 - and those guys were a pretty productive group.
Conclusion
So, nuclear weapons? Bad. Science? Hard. Scientific progress? Slower.
The solutions to each problem are pretty nuanced, of course, but one could take a look at development economics and try to develop an approach that prioritizes innovation and experimentation versus the same old, same old.
There is some nuance here, because studies also find that most scientists’ most important work is later on in their careers, so it might just have something to do with spending less time doing research and more time in grad school.
I read American Prometheus several years back and it took me several sentences to realize that "El Alamo" was Los Alamos. Is this an Argentinian translation?