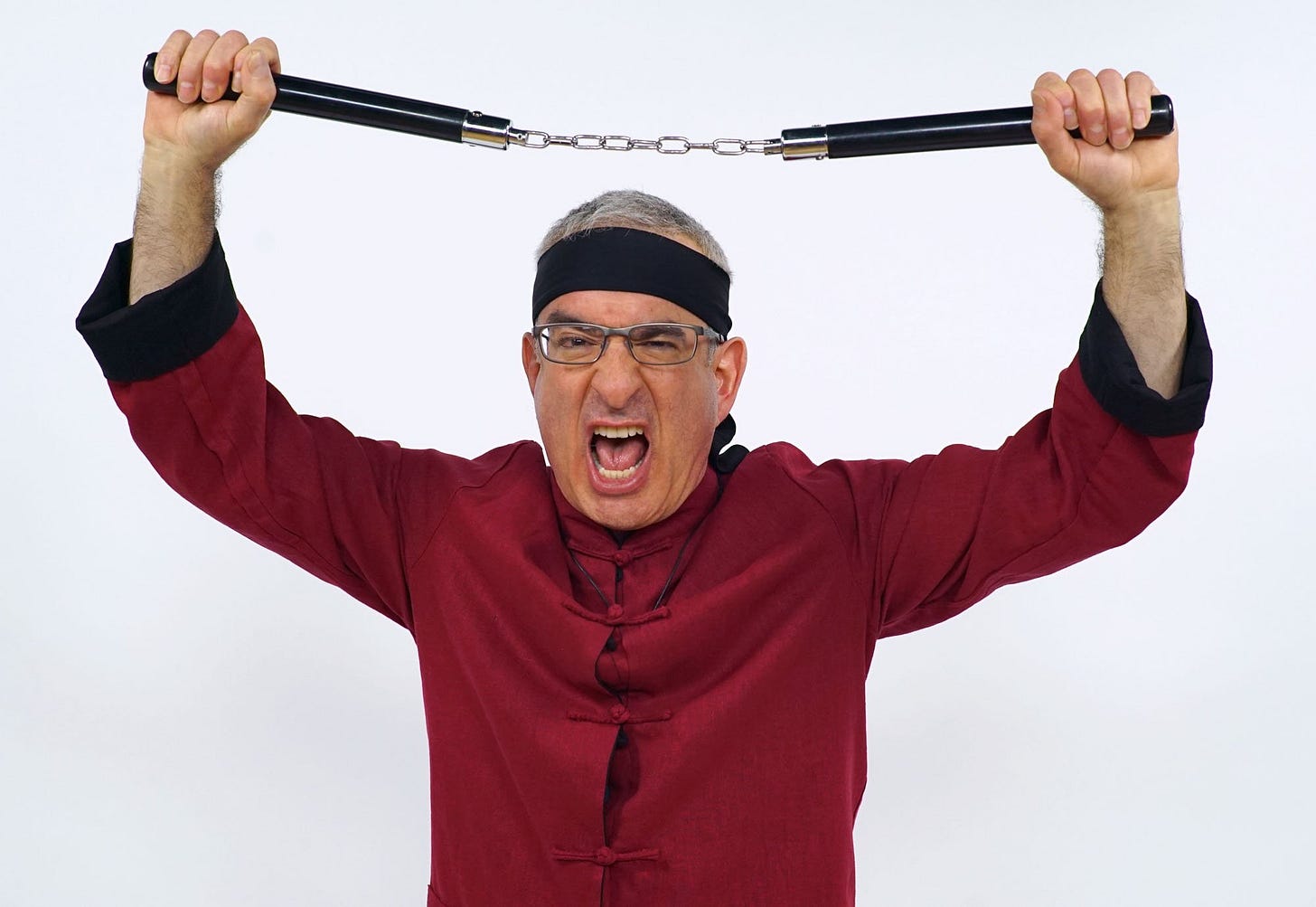
Econometricians, they’re ever so pious / are they doing real science or confirming their bias?
Fight of the Century: Keynes vs. Hayek - Economics Rap Battle Round Two
After talking about whether or not economics is scientific, the limits of knowledge economic rationality itself places on us, and about the struggle to isolate causality in economics, let’s look at one final issue: is data enough? What data does economics use? And how does it use it?
What is econometrics?
In most circumstances, economists don’t have access to experiments, so the way to acquire and use data is by looking at already collected statistics and drawing inferences from them. But how does econometrics work?
In very broad terms, you use a set independent variables (called “x” in general) and a random exogenous term (the error, “u”) to explain an independent variable (“y”). The dependent variables represent observable causes, the independent variable outcomes, and the error term stands in for unobservable variables and exogenous shocks. There is a fixed effect, and the marginal effect of each dependent variable are called “betas” (because of the Greek letters used).
The effects of each marginal variable are only causal in the abstract, but for an estimate of the model they can be flawed in many ways. To ensure that the estimated betas are closest to reality, econometricians use some assumptions, and the model can occasionally be “fixed” if some of them aren’t met. Time series econometrics has major problems because time obviously plays a role here, so instead it uses different ones that are also (potentially) fixable, at times. If you’re interested in more detail, pop open an econometrics textbook.
Using various mathy methods that aren’t relevant (just read Wooldridge’s textbook if you’re so interested), you can estimate the betas and quantify the effect. You also can run tests to determine whether the effects you’ve found are statistically significant, i.e. whether (one of the) dependent variables is relevant to explaining the independent variable. The key fact is that determining the causal links to study empirically has to be done through economic theory first.
Obviously you cannot rely on theory alone - a purely theoretical approach could reach any obviously false results by just assuming literally anything. And you cannot use evidence alone, since there are questions that can obviously not be answered empirically: which variables are causes and which are effects? What’s the best way to measure a specific variable?, etc. Without empirical evidence, someone could say that X causes Y, and someone that Y causes X, and even with evidence, unless they do very specific things, one could claim beta was 0.5 and the other that it was 2- and they’d both be right, and both be wrong.
Stone Age Econometrics
This is a sad and decidedly unscientific state of affairs we find ourselves in. Hardly anyone takes data analyses seriously. Or perhaps more accurately, hardly anyone takes anyone else’s data analyses seriously. Like elaborately plumed birds who have long since lost the ability to procreate but not the desire, we preen and strut and display our t-values.
So here comes a key concern for econometricians: how can you use econometrics to actually prove something if it’s possible to just find any correlation you want? The question of how to actually prove a causal relationship in empirical evidence is really important. It’s worth pointing out that no data is truly random (even medicine, the source of the gold standard RCT, had stuff such as thalidomide to contend with) and some sciences don’t actually perform any experiments and do just fine (astronomy). The question is more how to use data to prove causality.
The traditional way of doing is was, basically, to just run a regression and not worry too much about anything. One regression showed that inflation increases investment, another that it decreases it, both sides just murmur and say “identification” a lot, the issue isn’t brought up again. While there are thousands of data series available, only a handful have more than 50 or 60 years of data - and there are infinitely many possible specifications for their relationships. Econometrics back then was in such poor shape that it was the source of endless ridicule “if you torture data long enough, Nature will confess” (attributed to Ronald Coase), or “there are two things you are better off not watching in the making: sausages and econometric estimates”. Running regressions on the crime rate shows that, depending on how many variables (and how they are specified) you include, shows that the death penalty either increases, decreases, or doesn’t affect the rates of various kinds of crimes, or all crimes in general.
When you run a good old fashioned regression, you get the correlation coefficients, not really causality. This can be a problem for any number of reasons, primarily endogeneity (i.e. that the independent variables aren’t actually independent from unobservables), a form of endogeneity called simultaneity (that Y and X influence each other), and the fact that maybe there’s something else at play (omitted variables). Imagine education: people with more education earn more money. But does education play a role, or do they, say, have more talent (so they get more schooling), or have higher incomes and better connections, or education is completely worthless but getting more and better education makes you more employable? Traditional econometrics is particularly ill-suited to answer this.
Like something is brewing and ‘bout to begin…
Does the minimum wage reduce employment? Does immigration reduce employment? These questions are pretty simple to answer with theory: if the labor market is perfectly competitive, yes. If it’s not, no. But are these predictions true? That’s much, much harder to answer, particularly because changes in minimum wages and immigration are not exogenous to labor market conditions. Imagine a world where they always kill jobs. Then, politicians would allow more immigrants during booms and fewer during busts, and similarly for minimum wages. So traditional econometric estimates will never tell you whether minimum wages kill jobs or not.
Where does evidence come from? In general, there’s two kinds of data points: observational and experimental. Observational evidence is data that is created “by the world”, so to speak, and is generally not very good: you can’t really control for a number of problems, you’ll never be sure if your model captures any of the real effects happening, and the available data itself could be a potential minefield. You can get observational evidence for any number of pseudoscientific claims, like flat-eartherism. The use of observational evidence is basically a last resort, and someone like Friedman would advocate using it only to falsify a theory, not to draw out causal claims. Experimental data, on the other hand, comes “from a lab” and is the gold standard: it’s a little world that the scientist controls completely. But there are very few fields of economics where experimentation is possible, let alone moral, so it’s not perfect. Experimental data is superior because it’s replicable (usually), clearly captures the direct effect, and the results aren’t usually up for debate: either the thing happened, or it didn’t. Problem is, economists don’t have labs (usually), so they have to rely on observational data, and it’s subject to all the problems mentioned.
However, it is still possible to answer this. Imagine there are two cities right next to each other, with generally similar economies. One of them raises the minimum wage, and the other doesn’t. Using some simple techniques from traditional statistics, you could still produce a reliable estimate of whether or not employment went down, as long as both cities are similar enough. The now legendary Card & Krueger (1994) paper did this, by looking at a minimum wage increase in New Jersey and comparing restaurants in the border area between New Jersey, which raised the minimum wage, and neighboring Pennsylvania, which didn’t. Subtracting the change in Pennsylvania from the change in New Jersey, you can get a generally strong estimate of the effect of the policy (the differences-in-differences approach, originating from medicine). Their conclusion, shocking almost all their contemporaries in labor economics, was that there was no evidence that it did. This was not uncontroversial1, but eventually similar studies found consistently similar(-ish) results.
The general gist of it is the use of “in-between” quasi-experimental evidence: observational data that, for reasons left to chance, is very close to being experimental. Something like a natural experiment comes into play here: another good example is Angrist & Krueger (1991), which ponders if education improves earnings. Because so much of it is endogenous, you need a really good naturally occuring dataset - in this case, people who were born later into the same cohort, so they got less schooling because they graduated at a lower same age. The kids who were born later got less education, and it turns out they have lower earnings - since there’s no reason to expect being born a handful months apart to be very correlated to many other variables, you could say the sampling is random, so you’re capturing the full effect.
A Revolution you can believe in
Besides a more careful use of quasi-experimental data, is econometrics actually improving? Yes, actually, and a lot - the con has been mostly taken out.
Firstly, for a number of reasons (mostly the passage of time), there is much more data available than in the 70s, and of much higher quality. Additionally, improvements in econometrics rendered many of the previous sources of debate (like heteroskedasticity, serial correlation, or functional form) moot in almost all ways. Thirdly, the use of natural (and occasionally lab) experiments have provided much better sources of data than previous observational evidence. Fourth, new methods such as instrumental variables, regression discontinuity, and the aforementioned diff-in-diff allowed for more precise treatment of causal effects.
Instrumental variables serves the purpose of getting rid of endogeneity (i.e. that a variable is correlated with the error term, or other variables) - to do so, it’s possible to “replace” the endogenous variable with an instrument that helps explain it but not the rest of the model - say, trying to estimate the impact of enlisting in the military on lifetime earnings by using being drafted to Vietnam as an instrument (Angrist, 1990)2. An extension of the IV methodology estimates the effect of an intervention that has heterogenous responses - for example, being offered (or not offered) a class on how to use computers. Some of the people offered the class would have taken it anyways, and so would have people that weren’t offered - and some people in both groups wouldn’t have regardless of the offer. Under very light stipulations, it is possible to determine the impact of people in the treatment group (aka people who were offered the class) who wouldn’t have taken it - that is, it’s possible to use quasi-empirical or even observational data and get conclusions as precise as those in a clinical trial. This effect estimate is known as the Local Average Treatment Effect (or LATE), and while it’s not as good as experimental data, it is much better than a regression coefficient. Regression discontinuity is another technique related to IV that estimates whether something has a statistically significant effect by looking at samples where, for idiosyncratic reasons, there are big cutoffs between two values. For example, to measure whether class size has an impact on education outcomes, you can look at Israeli data (Angrist & Lavy, 1999). In Israel, class sizes are capped at 40, so classes with 41 people get split in two, and classes with 39 are kept together. If you look at similar enough classes, then you can estimate the impact of class size by comparing the test scores of size 41 cohort with the ones of size 39, since that’d be the main relevant difference - and class size.
But the methodology is not without its critics: because of the small scale of the questions it answers (try applying regression discontinuity to tax rates), the weakness of some of its answers to previous lines of debate, the fact that even actual randomized clinical trials contain confounding variables, bad choice of instruments, and overreliance on asymptotical properties.
Ch-ch-ch-changes
As in the Stones’ Age, well over half of the material in contemporary texts is concerned with Regression Properties, Regression Inference, Functional Form, and Assumption Failures and Fix-ups. The clearest change across book generations is the reduced space allocated to Simultaneous Equations Models.(...) Some of the volumes on our current book list have been through many editions, with first editions publishedin the Stones’ Age. It’s perhaps unsurprising that the topic distribution in Gujarati and Porter (2009) looks a lot like that in Gujarati (1978).
Angrist & Pischke (2010), “The Credibility Revolution in Empirical Economics”
What changed between now and then? Obviously, methodology. But the approach fundamentally taken by economists to empirical work has also changed, shifting away from placing the onus of finding causality on theory and then simply estimating the effect (or, in more sophisticated cases, verifying the theory) through econometrics.
The shift away from this approach, fundamentally, could be understood as simply a continuation in methodologies, but the truth is that the Credibility Revolution is, fundamentally, a development towards the “Friedmanite methodology”, as Lucas put it. Starting in the 70s, there has been consensus that you cannot assume that the causal relationships shown in the data remain constant in magnitude across time. If individuals are even remotely similar to rational, they respond to changes in policy and circumstances - so any beta hat from one dataset is basically worthless when new data is added. Unless empirical work can be somehow used to find causality, there is no way “Stone Age metrics” can provide any causality - “if you torture the data long enough, Nature will confess”.
More complex models based on fluctuating, unstable relationships also means more of an emphasis than ever on endogeneity and on credible research design. Having one data set, a few instruments, and an OLS regression with a long list of sophisticated controls is far less illuminating, and far more fragile, than simply having two groups that are roughly equal on average and comparing a change that affects just one of them. Methods relying on such comparisons are far simpler and, despite their limitations, far more robust to scrutiny.
Of the many criticisms of new techniques, of course, the technical ones are the least interesting to discuss, and the ones about questions are the most important ones. Critics of the Credibility Revolution say that, while it has allowed economists to confidently answer many more questions, they’re all much smaller ones. This is a worthy trade-off, in my opinion. However, it’s also interesting to ask if all the questions answered are worth asking, and in my opinion, they aren’t. Just because you can actually answer a question doesn’t mean you have to.
Conclusion
Is this good a good thing? Yes, it is, a very good one. For the longest time, the limitations on the empirical possibilities of economics have held the field back. Nowadays, however, a much larger sample of possible research agendas can be undertaken, with massive implications for both knowledge and for policy. The revolution in empirical economics has mostly been focused on microeconomics, and most fields are more or less utilizing the new techniques - until fairly recently Industrial Organization was the odd one out. Macroeconomics has a lot more of all the problems mentioned, but in general macroeconomists have spent the better part of the past two decades attempting to incorporate more empirics to their work.
The new agenda has had tremendous effects on policy as well, with an increased focus on what works versus what feels like it does. Ever since a number of ground breaking studies from Latin America, it’s become as consensus that cash transfers don’t discourage work as it has that the minimum wage does not reduce hiring, and policy circles have mostly shifted to discussing the boundaries of such estimates and specific qualifiers rather than “in principle” affairs. These are all significant changes that will in no doubt change the lives of a significant number of people.
There have been some major drawbacks too. Firstly, the limited external validity of experiments and other new data might mean that policies that only work in specific circumstances might be generalized. Secondly, while the “con” has been taken out of econometrics, perhaps the econ has too - empirical work has sprawled into fields that are completely outside the purview of what economics would have traditionally concerned itself with, much to the annoyance of actual experts on those fields. The focus on narrow phenomena and the limited external validity might mean that “big questions”, such as the fundamental causes of growth, are sidelined in favor of smaller, easier to experiment with, but ultimately less impactful areas.
The denial to economics of the dramatic and evidence of the “crucial” experiment does hinder the adequate testing of hypotheses; but this is much less significant than the difficulty it places in the way of achieving a reasonably prompt and wide consensus on the conclusions justified by the available evidence. It renders the weeding out of unsuccessful hypotheses slow and difficult. They are seldom downed for good and are always cropping up again.
All in all, I think that the turn towards experimentation, empirical work, and rigorous verification of claims is a net positive. Making better predictions, choosing better theories, and recommending better policies is both a long-term improvement for economics as a discipline and for society as a whole, since economic policymaking is a major source of both wellbeing and misery. Rigorous econometric work has been criticized from those who dissent on conclusions from both the left and the right, but fundamentally facts don’t have a bias - they’re facts.
Sources
Stone Age Econometrics
Leamer (1982), “Let’s Take the Con Out of Econometrics”
Friedman & Schwartz (1991), “Alternative Approaches to Analyzing Economic Data”
Lucas & Sargent (1979), “After Keynesian Macroeconomics”
Natural experiments and quasi-experimental data
Royal Swedish Academy of Sciences, “Scientific Background on the 2021 Nobel Prize in Economics” (popular background version here)
Alex Tabarrok, “A Nobel Prize for the Credibility Revolution”, Marginal Revolution, 2021
Douglas Clement, “Interview with David Card”, Minneapolis Fed, 2006
Peter Walker, “The Challenger”, IMF, 2016
Dylan Matthews, “What Alan Krueger taught the world about the minimum wage, education, and inequality”, Vox, 20193
The Credibility Revolution
Noah Smith, “The Econ Nobel we were all waiting for”, 2021
Leamer (2010), “Tantalus on the Road to Asymptopia”4
The Economist, “Cause and defect”, 2009
In a 2006 interview, Card stated: “I think my research is mischaracterized both by people who propose raising the minimum wage and by people who are opposed to it. What we were trying to do in our research was use the minimum wage as a lever to gain more understanding of how labor markets actually work …”
To be fair, this is not an uncontroversial finding, since most deployed to Vietnam were volunteers, and many people who had “high likelihood” draft numbers enlisted voluntarily anyways. It is unlikely that the “pure volunteers” and the involuntary draftees were similar, so applicability is limited to “pure draft” situations. Similar studies have been done about the impact of Argentina’s draft on crime rates.
Alan Krueger, a long time collaborator of 2021 Nobel Laureates David Card and Joshua Angrist, passed away in 2019. It’s likely he would have received the Prize last year.
The man has some talent for naming papers, I’ll give him that, in spite of his cranky libertarian views and bizarre political career.
A phenomenal breakdown of how econometrics has--and continues to--evolve. Could not agree more that though economists are moving towards answering smaller questions, but more precisely.
Two questions, and I’d love to hear what you think:
1) Do econometricians have a rather paradoxical issue where the complexity of their math that arrived at a certain conclusion paints their world-view too rigidly, to the point where they are unable to identify the obvious at times, or present a false sense of certainty (Though I suppose that the “obvious” is subjective.)
2) Are you on Twitter?!